University of Wisconsin mechanical engineering students are developing and testing a digital insect light trap that will use low-cost ultraviolet LEDs, cameras, and micro-computers to assess insect populations cheaper, faster, and better than current methods. Dubbed the Stream Night Life, this device will be used to measure the health of streams and other aquatic and terrestrial ecosystems by monitoring the insects that are drawn to the traps.
The Need for Insect Population Data
Aquatic insects and other invertebrates are key links in both terrestrial and aquatic food webs and sensitive indicators of environmental health. Both angler observations by fly fishers and scientific studies indicate aquatic insect species diversity and populations are declining. While there’s debate among scientists regarding the magnitude and rate of these declines and what factors may be most responsible, there is little disagreement that the amount of aquatic and terrestrial insect population data currently available is woefully inadequate to document spatial or temporal insect population trends.
For example, the Wisconsin Department of Natural Resources collects about 400 aquatic invertebrate samples annually; that equates to about one sample for every 100 miles of streams in the state. These samples are processed at two state university labs, costing hundreds of dollars per sample and requiring 6–8 months for the resulting data. Only a few pest insect species, such as emerald ash borers and spongy moths, are tracked by state and federal agencies. Moreover, the U.S. EPA’s National Rivers and Streams Assessment collects aquatic insect taxonomic data on a national scale only every four years, and there are no systematic efforts to track adult aquatic insects. Better monitoring data are fundamental for improving our understanding of insect diversity and population trends in both aquatic and terrestrial environments.
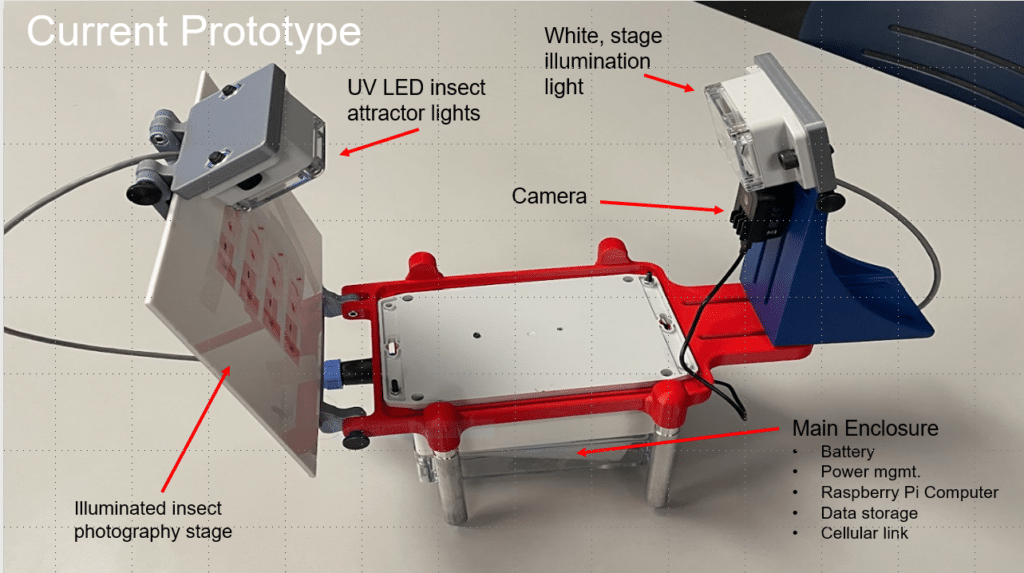
Previous Insect Light Trapping Method
For over 100 years, lighted insect traps have been used to assess insect populations. Traps are often comprised of white bedsheets suspended by a rope and illuminated with gas lanterns or electric lamps. During the night, entomologists either pluck the specimens of interest off the sheet or the sheet is draped in a tub of an alcohol solution to trap the insects drawn to the light. After the night’s trapping, entomologists then sieve the drowned insects out of the tub and begin the laborious task of sorting and identifying the specimens collected.
New Technologies
With global concerns about declining wildlife populations, new camera trap technologies are being developed to improve the assessment of animal species worldwide. Like trail cams, camera traps collect imagery data, but with the addition of microcomputers and artificial intelligence, the images and associated environmental data can be processed automatically in the field to identify and count the animals of interest and identify key environmental attributes of the animals’ ecology. Some of these devices transmit sampling data collected at remote field locations to the researcher’s office computers via cellular links. The DIOPSIS project in the Netherlands and the AMI-trap in the UK have developed camera-based insect monitoring systems.
Light Trap Operation
At night, the autonomous Stream Night Life will power up and photograph insects attracted to a stage lit by ultraviolet LEDs. The imagery data will be captured on an onboard microcomputer and then transmitted to a computer server via a cellular link. A primary goal of the project is to ultimately use artificial intelligence to automatically identify and quantify the flying insects drawn to the traps.
A major task in this effort will be the collection of the hundreds of photographs necessary to develop the computer algorithms used to identify and count the insects. Images of individual adult aquatic insects will be identified, labeled, and fed into a Deep Learning program to train software to identify and count specimens. The resulting algorithm will then be used to identify and count the insects captured in subsequent photographs.
Seeking Collaboration
The plan is to design a low-cost, open-source, relatively easy-to-build device that can be deployed by a consortium of state and federal agencies, and private organizations like Trout Unlimited. Collaboration on crowdsourcing of the annotated images will help expedite the Deep Learning process. Initially, the insect taxonomic identifications will be relatively coarse, most likely at the Order level (for example, mayflies, caddisflies, stoneflies). Abundances and proportions of each of these insect taxa and how they differ over time and among locations can provide powerful information on the conditions of streams and other aquatic and terrestrial environments. Imagery data collected at stream sites will be compared against traditional aquatic kick net methods to assess how representative the light trap samples are of the entire assemblage of aquatic invertebrates found in streams.
The engineers are focused on advancing the device’s functionality and stability of the initial prototype built in 2023; a major structural redesign to improve field ruggedness and ease of deployment comes next. The device uses a Raspberry Pi to control the camera, lighting, data storage, and transmission. Additional refinements being considered include sensors for air temperature, humidity, and wind speed, given that these environmental factors strongly influence insect activity.
The students are working with stream scientists investigating which adult aquatic insect taxa are drawn to light traps, how representative these taxa are of entire stream invertebrate assemblages, and what biological indexes of stream health can be developed to interpret the trap data. A goal for this year is to build a more advanced prototype for summer field testing in 2025. The students receive guidance from University of Wisconsin Mechanical Engineering Professor Graham Wabiszewski and stream ecologist Mike Miller of the Wisconsin Department of Natural Resources.
Again, we welcome feedback (michaela.miller@wisconsin.gov) from those interested in collaboration in crowdsourcing of adult aquatic insect images, engineering feedback on the prototype design, or field testers of an advanced prototype in 2025.
Thanks so much for the posting. Fascinating. While R.Pi might be great for prototyping – it does require a lot of mW power, and it can’t be put to sleep. A great way of reducing total deployment costs is to have low total power consumption – one way to do that is to have a sampling window with all circuitry shut off in between sampling windows.
Are you considering adding a powering section to your analysis, that is including in the type of computing platform with the powering to consider the total cost of deployment.
There are other options for processor/powering a camera – and it may just be that the mechanical computing section allows for different platforms. .
The other issue I’ve seen with cameras I’ve got, is that spiders take a random walk – including across camera lense. It might need that there is some kind of lens clearing capability – a blink so to speak. Could be brush. Look forward to hearing more.
Neil, thanks for your input. My background is in stream ecology and the engineering students I have been working with are providing the technological expertise that I certainly don’t have. I know the students have been discussing the use of Pi Zeros I believe for the same power management issues you’ve touched on. Perhaps because I am not an engineer I am not grasping the significance of your following statement. “Are you considering adding a powering section to your analysis, that is including in the type of computing platform with the powering to consider the total cost of deployment.”There are other options for processor/powering a camera – and it may just be that the mechanical computing section allows for different platforms.”
Simpler issues I can grasp; I have been contemplating cycling the trap on and off during the night to among other things get insect attracted to the trap to disperse, which may be of value in better characterizing the local insect assemblage by having multiple trapping events per night and may help with power management. I also was wondering about how to clear insects from the stage we hope to attract the insects to, perhaps with e.g. a small fan. I had not considered what to do if insect begin camping out on the camera lens itself. The students I am working with are a bright bunch but lack (as I) any field experience with such devices and welcome any guidance you or others can provide. Thanks for your time. Mike
Hi @Mike Miller, good to have a variety of skills, and the target of making stuff/engineering is to have a customer or user -your 🙂 and then the user phrasing requirements – often iteratively with the engineering side.
I also volunteer at the local uni as industry advisor for their “Capstone Engineering Program”. For capstones projects, its often about the challenge of getting started- teaching basics and team work. Phrasing a requirement, then taking it through design and verification testing it, and then a poster to explain it. Often getting it all actually working or capturing enough of it for another group to start on following year – is a big challenge, learning curve – and not on the marking chart. Real world users, appreciates a working prototype.
Good to hear that powering is on the list – at the level power could be in real basics – deploy by a stream, leave for six months and then return to pick up. That could be 3 D cell batteries, or Solar Panel 3W with 4AHr battery – and/or could be statistically take camera shots as power saver. Of course if you have a camera – then I assume there is visual recognition computing algorithm – which requires a certain size of processor. Or maybe its simpler – just take 5 pictures 1sec apart every 15minutes, and store on a uSD, and analyze later.
I wonder if the audible signatures that most winged insects leave would be sufficient ID. Of if the buzz can be decoded by a computer sci project to specific insects.
There is a low cost device called Audio Moth that is purpose built for this and maybe of interest – https://www.openacousticdevices.info/audiomoth
I think there is an open source version – and depending on what type of student course it is – it maybe could have a software modification to add a LED that gets turned on periodically to attract insects to near the microphone.
For the aspect of visual analysis – perhaps one of these hardware modules might be appropriate
https://docs.edgeimpulse.com/docs/edge-ai-hardware/edge-ai-hardware
Which partly means choosing the size of a camera, seems like its is 2Mpixel or 5Mpixel
https://docs.edgeimpulse.com/docs/edge-ai-hardware/mcu/openmv-cam-h7-plus
There is also a discussion of noise analysis – could different insect noise signatures be used
https://www.hackster.io/c_m_cooper/monitor-your-noisy-neighbours-with-edge-impulse-9e5edd
Hello Neilh20
Sorry for the slow response. As mentioned as an aquatic ecologist I am more on the end user side of the insect camera trap development than the front-end design and am grasping about 40% of the significance of your commentary. Regarding power demands of such a device I think the deployment (at least initially during testing) will be several days at most, with theft and tampering being some of the concerns for longer deployment. Regarding use of insect sound (wing beats) to ID various insects, I am aware of at least one company that is using sound for their insect identification, but as I understand it, they are trying to identify maybe 3 or 4 insect pest species in crop fields that are relatively species-poor versus a much more diverse assemblage likely to be encountered stream-side. Also, I have no idea if the sounds of individual insect taxa can be isolated in a potential chorus of insect wing beats that might occur in more natural environments. Lastly, as I dimly understand, for AI training (at least with imagery data) the images need to be annotated with the name of insect in each image to train the model, and off the cuff am not certain how that would be accomplished with sound. With the school semester winding down rapidly, unfortunately the engineering students will scatter for the summer and insect design advancements are likely to stall-out. Mike
Its some good thinking and takes time to scope it. From what I understand, some of the AI on vision detection may have a lot of promotion without understanding what it takes technically to make it reliable. I’ve tried with a few simple realworld items on a student project – simple pedestrian/bicycle counter. The challenge is also false positives. It could be that the first pass is a device that takes pictures and stores sound and need to be person processed.
Pictures are complex, and I hear sometimes it can take a lot to train algorithms on them. Sound may actually be simpler to decode – One example is the Merlin App on birds. Its fascinating to watch Merlin visualize the sound and then how much it decodes.